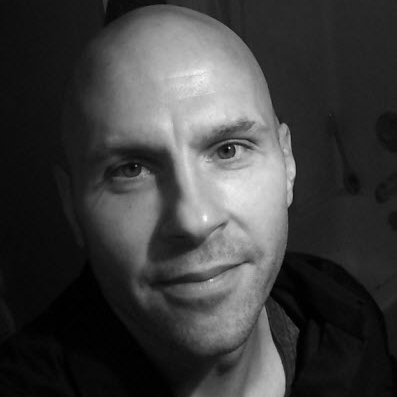
Contact Information
- Daniel J. Denis
- Skaggs Bldg 369
- Email: daniel.denis@umontana.edu
- Office Hours:
Dr. Denis is on sabbatical for the 2023 -2024 academic year
Education
B.A. (1997). Laurentian University, Sudbury, Canada. Thesis: The power of suggestion and context effects in the production of overt behaviours.
M.A. (1999). York University, Toronto, Canada. Thesis: Null hypothesis significance testing: History, criticisms, and alternatives.
Ph.D. (2004). York University, Toronto, Canada. Dissertation: The Rise of Multivariate Statistics.
Courses Taught
Psyx. 222: Psychological Statistics (UGRAD)
Psyx. 297: Independent Study "Teaching and Tutoring Statistics" (UGRAD)
Psyx. 520: Advanced Psychological Statistics (STAT I) (GRAD)
Psyx. 521: Advanced Psychological Statistics (STAT II) (GRAD)
Psyx. 522: Multivariate Statistics (GRAD)
* The following are possible independent studies that I would be willing to supervise or develop more extensively into a course. If you have an interest in any of the following topics, please feel free to contact me (students outside of the psychology department are also encouraged to contact me for mentorship opportunities):
Psyx. 629: Practicum in Statistical Teaching and Consulting in the Social and Natural Sciences (UGRAD/GRAD)
Psyx. 629: Generalized Latent Variable Modeling (UGRAD/GRAD)
Psyx. 629: Quantitative, Historical, and Philosophical Foundations of Scientific Practice (UGRAD/GRAD)
Psyx. 629: Data Visualization (UGRAD/GRAD)
Psyx. 629: Reconciling the Humanities with STEM (UGRAD/GRAD)
Psyx. 629: Philosophical Psychology (UGRAD/GRAD)
Field of Study
My interests are in the areas of teaching statistics and research methodology in the psychological, social and natural sciences, critical analysis of their use in scientific practice, and effective yet still rigorous communication of statistical concepts to students of science and practitioners through demystifying otherwise seemingly complex subjects. This is done through emphasizing foundational and unifying principles along with examples drawn from scientific practice (e.g., the COVID-19 pandemic). The fundamental goal of all education, whatever the subject, should be to help students understand in their personal quest for a widening and deepening sense of awareness. My teaching philosophy is to help and challenge students to think on their own so that they become independent and critical consumers and producers of knowledge. I sincerely want students to understand what they are learning. Ask me a question and you will get another question in return (closer in proximity to the answer you seek) because I want you to build up the knowledge for yourself and make it "yours." You already own the answer, you just need guidance on the path that will eventually uncover it. Providing you with quick answers, without you working on the problem yourself, accomplishes nothing for you other than the illusion of having learned something. Good education faciliates you in your personal quest. Good education empowers you to find your own answers. Mentorship helps you figure out your own, individual path. My goal for you is to become an independent learner, evaluator, and producer of knowlege (empirical knowledge or otherwise), help you appreciate what you know, but also help you understand how much there is yet to learn. Humility in knowledge is mandatory for learning. Humanity, as a whole, knows well less than 0.0000001% of all there is to be known. In my courses, I encourage students to bring their proposed understanding to the table to receive feedback instead of simply asking a question without providing a starting point of where they are currently at in terms of their own understanding. When it comes to learning, the ball has to be in "your court" (most of the time) so that you build up understanding for yourself. This helps to generate confidence in what you do learn, and sets you on a path to building your own foundation, rather than simply "regurgitating" what you are learning.
Evaluating Scientific Evidence
Good science is about challenging the status quo, not blindly submitting to it. To use a COVID-19 vaccine analogy, if you can critically evaluate a research report from multiple angles, subject it to healthy scrutiny, and it is still "standing" after such evaluation, then it may indeed be something you wish to inject into your arm! But without critically evaluating a research claim (whether in biology, psychology, forestry, etc.), you simply will not know whether the research product has any merit, and will simply buy into whatever claims are made by the authors. Virtually all research results "go through" design and statistics, and so understanding statistics very well is crucial for the student who wants to understand (and critically evaluate) scientific literature. As another example, without understanding and appreciating the assumptions (both technical and philosophical, where "philosophical" translates to "very rigorous," usually an even deeper rigor than the technical representation using symbols) underlying factor analysis and latent variable models, for instance, one is at a deficit to understanding what is being communicated by a research report that uses the technique. Without appreciating issues of omitted variables and non-uniqueness of loadings, for example, one is simply not in a position to truly appreciate the published factor analysis appearing in a journal. Beyond that, an even richer understanding of a statistical technique (or most other things) can be achieved by exploring its historical foundations (which includes technical, social and political), though I do not usually teach these historical elements (at least anywhere extensively) in my mainstream coursework featuring applied statistics in the sciences.
Quantitative vs. Qualitative Approaches
I equally value quantitative and qualitative approaches to knowing, and hold the humanities in very high regard, especially analytical and critical approaches in both philosophy and history. My current views are that most elementary mathematical objects are the extensions of concepts, and hence their "underground" is much more loosely defined. Continuity, for instance, is nothing more than a rigorized version of an "idea" human beings have probably had forever. True continuity exists nowhere in the real world, but rather is an idea simply of our minds. The "rigorous" definition of continuity (e.g., epsilon-delta) is merely an attempt (weak and imperfect at that) to communicate these ideas or concepts, similar to the "idea" of beauty, for instance, but for which a "rigorous" (read: "surface") definition does not exist, and therefore is more difficult to grapple with. For example, you can express continuity with a (relatively) simple definition, but you typically need to read a book in the humanities to get even a glimpse of what the idea of love might be. Both love and continuity are based on underlying concepts and essences, but one is much more shallowly "rigorized" than the other. It's one reason why the objects of mathematics might be considered more simply defined (relatively speaking) than constructs in the humanities, for instance. All considered, symbolic representation, whether in symbolic languages or the humanities, might be considered among the weakest forms of expressing a deeper concept that first originated as a "mushy" ill-defined idea (e.g., consider that the idea or concept of correlation has existed probably forever and only became "rigorized" with Galton and Pearson in the 1890s). But for the purpose of communication, it helps, and makes the mathematical sciences exceptionally powerful and useful (and envied) both on their own merit, but also in describing our world. The humanities are equally as powerful as STEM, yet working with and communicating about its objects of study, if attempted rigorously, can be even more challenging, or seemingly lead to no progress at all at times due to this inherent difficulty in defining and communicating about the objects under study. Likewise in the psychological sciences, truly capturing ideas such as self-actualization, depression, dissonance, can be exceedingly difficult and challenging, regardless of attempts using psychometric instruments or clinical assessments.
In my own studies and pursuits, I have been led primarily to statistical and methodological areas for their emphasis on rigor and precision. However, history and philosophy can be even more challenging when approached analytically. The "pieces" are different, but the analytical requirements are just as demanding. For example, statistical reasoning is based on fundamentals of logic, which is philosophy, and appreciating where correlation "came from" is history. Hence, in my writing, and to some extent teaching, I often combine features of all these areas to better communicate concepts and principles. Students and readers often appreciate learning things from "first principles," (a "ground zero" approach) as it then makes it easier for them to own the concept themselves, instead of feeling like it was something arbitrary dished out to them in a textbook without any foundation. Textbooks usually contain the "tip of the iceberg" only. The "underwater" is in the history and theory of how those concepts evolved. Education shouldn't be about uncritically memorizing a bunch of stuff from a book, regurgitating it on a test, and then pretending as though the material has been somehow grasped. It should be about critically understanding those elements learned, how they relate to one another, how they arose to some extent, and even how they are lacking. Learn to evaluate things on their own merit. Learn to see things "anew" even if you've seen them many times before. The new appraisal on already-learned material may offer new gems and insights and allow you to grasp them at a much deeper level than previous. Education should help make you think carefully about what you are learning now, and even more importantly what you will learn in the future as you move on with your career and interests. Education helps you develop the tools to learn, and teaches you how to distinguish truth from falsity in a variety of settings. Scientists employ an empirical philosophy, and it is essential that you are able to assess whether the conclusions emanating from that empirical approach (or any other) are in agreement with the methodology employed. In all of my teaching (and most of my writing), I strive to help students "get to the bottom of things" as they are confronted with a myriad of methodology, statistics, and in some cases distracting (and potentially unethical) "scientific marketing."
Book Publishing & Recent Directions
For the better part of the last 10 years (since 2012 when I received my first book contract with Wiley), I have been authoring books on applied statistics for the social and natural sciences featuring R, SPSS, and Python software. The most significant and thorough (and the one I'm most proud of and which took an inordinate (mildly put) amount of time and effort) of these projects is Applied Univariate, Bivariate, and Multivariate Statistics: Understanding Statistics for the Social and Natural Sciences with Applications in SPSS and R, now in its 2nd edition (2021). This book combines a mix of theory and application, but also philosophical, historical, and ethical context (i.e., products of my own research and thinking of these issues) regarding the various methodologies surveyed to help the user know what can vs. cannot be concluded scientifically from the application of a given technique in conjunction with experimental vs. non-experimental design and other scientific issues. The other three books (on R, SPSS, and Python) are smaller introductory beginner books on applied statistics that feature the chosen software in data-analytic demonstrations. I was recently invited to contribute a chapter to Robert J. Sternberg (of "Sternberg's IQ") & Wade Pickren's The Cambridge Handbook of the Intellectual History of Psychology. The chapter was one on the history of methodology and statistics in psychology, and was co-authored with my graduate student Briana Young. Receiving an invitation to contribute to this volume was among my greatest honors as an author to date.
The historical evolution of statistical progress occurs in the context of a wider zeitgeist, and conceptual seedlings predate rigorous definition by sometimes thousands of years. This has been well established by historians of statistics and science. Understanding how quantitative methods evolved as a way of mapping the "real world" or whether they evolved independent of practical considerations is an interest of mine, as well as what can vs. cannot be ethically concluded from a scientific investigation that employs such quantitative tools. Too often, scientists overestimate the power of the analytical tool in supporting their scientific hypotheses. In my teaching, I aim to help students understand and appreciate just what can vs. cannot be concluded from the use of statistics in a research article. In this sense, my teaching encourages students to think independently and critically when confronted with science that uses statistics, and to look at the "Big Picture" when interpreting a research article. Assumptions, methodology, design, statistics, psychometric properties of variables, these are all factors that need to be considered to arrive at the "bottom line" of what a research report is communicating, which does not always coincide with what authors would like you to take away from the research. Critically evaluating research does not imply being "negative," or "pessimistic," it simply means trying to accurately assess what can vs. cannot be ethically gleamed from a research finding. Design, not statistical analysis, is usually what is most important in establishing a scientific finding. Complex statistical analyses on an unstable design foundation is analogous to building a house on quicksand. The house may be impressive, but the entire structure will fail inspection.
Understanding how statistical models work and function is vital to understanding research that is driven by statistical methods. Understanding the fundamental statistical and philosophical foundations that precede the application of quantitative tools is paramount for understanding how the statistical methodologies are being applied in a given situation, and whether that application is valid and ethical. Mathematical statisticans (bless them!) and others have provided science with exceptional tools, and it is essential that students learn how to successfully (and ethically) incorporate that wealth of mathematics into their scientific endeavors and applications. Too often, this incorporation is done recklessly with insufficient knowledge of what the statistical method can vs. cannot tell you about your data.
CURRENTLY RECRUITING STUDENTS
Would you like to be trained in the rigorous understanding of how statistics are used in psychology and related areas, and what can vs. cannot be learned empirically and methodologically from their application through interdisciplinary knowledge of psychology, statistics, philosophy and history? If you have interests in any of the above or would like to get trained in quantitative methods in psychology at the UNDERGRADUATE or GRADUATE levels, would like to work in academia or corporate settings, (or for now would like to work on an independent study (UGRAD or GRAD)), please contact me at daniel.denis@umontana.edu or apply directly to the Experimental Psychology Program. In addition to academic jobs, there are many high-paying jobs in private industry that demand combined knowledge of human behavior, statistics, behavioral data science, psychometric measurement, etc. The following link contains some examples, including jobs at the Educational Testing Service and Google: JOBS. Below are just a couple samples of what the job market is looking for when it comes to combining behavioral science with quantitative expertise:
Full Job Description
- Category: Research and Sciences
- Location: Seaside, California
- US Citizenship Required for this Position: Yes
- Clearance Type: Secret
- Telecommute: Yes – May Consider Full Time Teleworking for this position
- Shift: 1st Shift
- Travel Required: No
- Positions Available: 1
Peraton has ongoing work with the Department of Defense to provide research and innovation to the Military Services. This research pertains to multiple areas, but may include Service member wellness, suitability for Military Service, evaluation of interventions, and implementation and evaluation of automated solutions to staffing, manning, and other human resource data systems. The individual hired for this research position will be required to do the following:
- Conduct research using behavioral science principles, methodologies, and technologies in an applied governmental setting
- Develop research designs, perform literature reviews, collect field data through interviews and focus groups, analyze existing databases, and conduct statistical or descriptive analyses
- Describe results of research through peer-reviewed publications, briefings and technical reports
- Work cooperatively with team members to ensure quality, timeliness, and accuracy of research products
- Interface with Military customers and consumers to meet their requirements, and assist in implementation of research findings and products
- Supporting the development of research designs
- Performing literature reviews
- Collecting field data
- Conducting interviews
- Administering surveys and conducting focus groups
- Data cleaning, extraction, transformation, and loading
- Statistical and qualitative analyses
- Collection and analysis of research materials to support technical reports and briefing materials
- Coordination with virtual research teams
- Personnel Security Clearances
- Personnel Placement and Suitability
- Suicide Prevention
- Sexual Assault Prevention & Response
- Espionage and Insider Threat
- Data Science
- Master’s or PhD degree in a Social or Behavioral Science field such as Psychology, Sociology, or Criminal Justice and minimum of 2 years of work experience in public health and an applied research environment.
- Minimum of 2 years’ experience with Project Management.
- Ability to demonstrate Strong Client Communication skills.
- Extensive quantitative analysis or advanced statistical analysis.
- Experience with statistical analysis software, to include R.
- U.S. Citizenship and the ability to obtain and hold a Secret security clearance.
- PhD in Social or Behavioral Sciences.
- Experience with personnel selection, background investigations, or personnel security vetting.
- Experience in leading research projects and mentoring team members.
- Experience with contract research.
- Experience with supporting/writing DoD proposals.
- Current, Active U.S. Government Security Clearance (issued within past 5 years).
“The yearly compensation range for this role is: Minimum- $103,680 to Maximum- $ 155,500. The successful candidate will be offered a yearly compensation that aligns with their individual skills and experience as it directly relates to the position requirements.
In addition to the yearly salary, Peraton provides a variety of benefits to include: health insurance coverage, life and disability insurance, savings plan, company paid holidays and paid time off (PTO).” We are an Equal Opportunity/Affirmative Action Employer. We consider applicants without regard to race, color, religion, age, national origin, ancestry, ethnicity, gender, gender identity, gender expression, sexual orientation, marital status, veteran status, disability, genetic information, citizenship status, or membership in any other group protected by federal, state, or local law.
Quantitative User Experience Researcher
Selected Publications
Denis, D. (2021). Applied Univariate, Bivariate, and Multivariate Statistics Using Python: A Beginner's Guide to Advanced Data Analysis. John Wiley & Sons.
Denis, D. (2021). Applied Univariate, Bivariate, and Multivariate Statistics: Understanding Statisics for Social and Natural Scientists, With Applications in SPSS and R. 2nd Edition. John Wiley & Sons.
Denis, D. (2020). Univariate, Bivariate, and Multivariate Statistics Using R: Quantitative Tools for Data Analysis and Data Science. John Wiley & Sons.
Denis, D. & Young, B. (2019). Methodology in Psychology. In Sternberg, R. J. & Pickren, W. E. The Cambridge Handbook of The Intellectual History of Psychology. Cambridge University Press.
Denis, D. (2019). SPSS Data Analysis for Univariate, Bivariate, and Multivariate Statistics. John Wiley & Sons.
Denis, D. (2015). Applied Univariate, Bivariate, and Multivariate Statistics. 1st Edition. John Wiley & Sons.
Denis, D., & Docherty, K. (2007). Late nineteenth century Britain: A social, political, and methodological context for the rise of multivariate statistics. Journale Electronique d’Histoire des Probabilités et de la Statistique, 3.
Denis, D., & Legerski, J. (2006). Causal Modeling and the Origins of Path Analysis. Theory & Science, 7.
Friendly, M., & Denis, D. (2005). The early origins and development of the scatterplot. Journal of the History of the Behavioral Sciences, 41, 103-130.
Denis, D. (2004). The Modern Hypothesis Testing Hybrid: R. A. Fisher’s Fading Influence. With Discussion by Michel Armatte, Bernard Bru, Michael Friendly, Jeff Gill, Ernest Kwan, Bruno Lecoutre, Marie-Paul Lecoutre, Jacques Poitevineau and Stephen Stigler. Journal de la Société Française de Statistique, 145, 5-26.
Denis, D. (2001). The Origins of Correlation and Regression: Francis Galton or Auguste Bravais and the Error Theorists? History and Philosophy of Psychology Bulletin, 13, 36-44.
Friendly, M., & Denis, D. (2001). The Roots and Branches of Modern Statistical Graphics. Journal de la Société Française de Statistique, 141, 51-60.
Professional Experience
2004-present - Professor of Quantitative Psychology, University of Montana, Missoula, MT
2009-2012 - Experimental Program Coordinator, University of Montana, Missoula, MT
2008-2009 Statistics Incremental Advantage Instructor, NY.
2005-2008 - Director of Statistical Consulting Laboratory in the Department of Psychology, University of Montana, Missoula, MT.
2003-2004 - Course Director Statistical Methods I & II, York University, Toronto, CANADA.
2002-2003 - Statistical Consultant, York University, Toronto, CA
2000-2003 - Statistics & Computer Advisor, York University, Toronto, CA